So now I'd like to introduce today's speaker.
Today's featured speaker is Olav Solgaard.
Olav Solgaard is the professor of electrical engineering
of Stanford University, and the director of the Edward L Ginzton Laboratory.
He earned his PhD degree from Stanford University in 1992, where his doctoral
dissertation was the basis for the establishment of a Silicon Valley firm,
Silicon Light Machines, also known as SLM, cofounded by Dr. Solgaard in 1994.
He was a postdoc at UC Berkley, and in 1995 he joined
the electrical engineering faculty of the University of California Davis.
His work at UC Davis lead to the invention of the multiwavelength fiber optical
switch, which has been developed into commercial products by several companies.
Professor Solgaard's research interests include optical MEMS,
photonic crystals, atomic force microscopy, and solar energy conversions.
He has authored more than 300 technical publications and holds 50 patents.
He is a fellow of The Optical Society of America, the Royal Norwegian Society of
Sciences and Letters, and the Norwegian Academy of Technological Sciences.
He also serves as the academic director for the new Internet of Things graduate
certificate offered through the Stanford Center for Professional Development,
which we will talk a little bit more about at the end of the hour.
So now I'd like to turn the floor over to Olav.
>> Thank you very much, Stephanie, and thank you to all of you out there who have
logged on to hear me talk about things that are very dear to my heart.
I've been working with sensors for a long period, a long time, so smart sensors
are something that I'm very excited about and have been for a long time.
The Internet of Things, this newer base also has changed how we look at sensors
quite a bit, and that's part of what I'm going to be talking about today.
So here's my outline, I'm going to talk a little bit about sensor fundamentals.
Because that allows you to sort of understand a little bit better how you
should design and operate sensors in the Internet of Things sort of environment.
And I will make the argument, and I hope I make a strong argument that MEMS
sensors, meaning sensors that are made with silicon foundary technologies.
And I'll talk a little bit about what they are.
All the ones that really are going to enable the Internet, and has been doing so
up till now, and will do it even more on that in the future.
And then I will, towards the end, once we have sort of understood what a MEMS
sensor is and what it does, I'll talk a little bit about Internet of Things and
how that is going to be enabled by MEMS sensors.
And I'll talk a little bit about the opportunities that I see.
And really inviting you to sort of think about what Internet of Things can be for
you in terms of challenges and opportunities.
So that's towards the end, but before I even jump into the center part of it,
I just wanted to say a few things about my perception on Internet of things.
And Internet of Things is showing here as a pink cloud,
has many components to it, obviously.
It is sensors, or I have the perspective of sensors,
and many other people look at tit from different perspectives.
So you might be an expert on the Internet and how you use the Internet.
Of course that's really the backbone that ties everything together.
Machine learning and signal processing at the far end is also going to be very
important, and many other opportunities and challenges are there.
Circuits and embedded systems are the things that sort of takes the sensors and
connects them to the Internet.
So you can think of it in this way,
the sensors are the structures that allows us to interact with the real world.
And then they take it all the way to the other end through the embedded systems,
through the circuits, through the Internet, through machine learning, and
signal processing into the digital world.
So the sensors are the interface, if you like.
And that's why I think they are the most important,
of course other people have different perspectives on that.
But here's some of the reasons why I think sensors are so important.
You use them just everyday, this is two very,
very incomplete lists that sort of sensors that you use every day,
involved with sensors are in your cellphone in particular.
In that you have a accelerometers in there, those are the ones that allows your
screen to rotate so the phone knows what orientation it is.
Gyros do some of the same things, also complements your GPS signals.
Timing circuits, microphones, light sensors, cameras, all these things,
heartbeat sensors, and proximity sensors.
The heartbeat sensor is the one that's fits in your Fitbit or
sort of device like that, that people more and more are using these days.
In your car you have many, many dozens of sensors, and
I'm sort of showing an incomplete list here.
The next thing I wanted to do was to talk about how sensors work.
And of course, if you look at this list, and
this is a partial list, you'll say, that's not a meaningful question,
because they obviously work in many different ways.
And so I'll try to find some commonalities so
I can say a few things about how sensors should work and how they should scale.
So let's take a look at some of the ones that are out there.
This is Standford work, this is a company called Si Time.
What they do is make clocks, essentially.
So these are clocks that are buried in your silicon chip.
And timing is, of course, super important when you do communications, obviously.
This is the type of stuff that sits behind everything you do.
You use it all the time, you don't even know it.
The really cool things about this particular clock,
if you like, is it work exactly like your grandfather clock at home.
It has an oscillator and
the frequency of that oscillation is what gives you the timing information.
Only this is a much more advanced thing and much more stable thing and
much better in terms of keeping time than your grandfather clock.
What we have here is, if you look at this piece that is sort of,
you can see as a cross section here.
That is a mass that is suspended on a spring.
And it's driven and resonance frequency,
we'll talk a little bit more about a resonance frequencies in a second.
But that needs to be very, very, well defined so
that you can get a very good time signal.
So this is a mass on the spring essentially.
Here's another example, this is a MEMS gyroscope.
There are several things I want to say about this,
this is a very complex structure.
The first I showed was from Stanford, this is from UC Berkeley.
I don't want to be too Stanford-centric,
although you might feel that I am already when I go through these sides.
This is from Berkeley, and the part that's the sensor is the gyro.
And so this is something that senses rotation.
So it's similar to the timing circuit I just shown in the sense that it's
essentially a mass suspended on a spring.
And that's the center of it, and
there is also a whole lot more going on here obviously.
Here's some of my work.this is an atomic force microscope.
So this allows us to send things down to the nanometer scale, and
maybe as little as 1 nanometer chip, more chip you get 10 nanometer resolution.
I show two different versions here, both of them have this same thing.
There is, if you look, there is a spring and there's a mass associated with
that spring, sort of at the end of the spring, and that allows us to vibrate.
If you look at the one the left, it sort of has a vibrating type of structure,
like a cantilever or a springboard.
The one on the right is more like a trampoline or something like that.
In both cases you have a spring and you have a mass suspended from it, and
that's part of the important operational characteristics of these devices.
Here's another one.
This is not really a sensor, but it's the type of stuff that could go into a LIDAR,
for instance.
This is a scanner, you see there's a mirror right there, and
that mirror is rotating, again, it's a rotating mass on a spring.
This is they will set in future LIDAR systems.
And we're really trying to miniaturize those to make them more efficient.
So LIDAR is the ranging system, an imaging system they use in self-driving cars.
Maybe the most famous example is the Texas Instruments projection technology,
they call it Digital Light Processing Technology.
But that is just a little aluminum mirrors, and
one of them in the center is ripped off here to show you the sort of substructure.
And that substructure is very interesting.
But again, it's a spring that sort of you can see those little white pieces.
Those are springs that connect it through the mirror, and
the mirror rotates on the spring.
Underneath there's all kinds of of other things.
There is integrated electronics in this particular chip,
and that's a theme that I will return to on occasion.
So again, you have this mass on this spring.
Here's some of my work going way back.
This is a grating light valve.
These are ribbons that move up and
down to create diffraction patterns that we can use to modulate light,
and we can also use them as sensors in different configurations.
This is again, so these ribbons have a mass.
They have a spring constant to carry masses on the spring.
So I think I'm trying to make the argument now that many sensors can be shown,
or can be through described in models, as a mass on a spring.
So now I'm going to show you a little bit about how that actually merge.
This is to give you a little bit of a feel for
how these type of sensors work, and also how they scale.
That's going to be my main point.
So here we see this is a very simple spring board that I
put together here on a.
This is actually a hacksaw blade.
If I engage it like that, you can see that it's a broad based at the frequency.
That's the resting frequency.
The resting frequency is the frequency where you have maximum energy storage in
terms of mechanical energy in the system.
Now it moves pretty fast here now, but it is already a sensory.
If I think of this as a sensor, right now of course it's actually an accelerometer.
It sits in a accelerating field and it's bent a little bit.
You can't really see it, but it's very much standing there,
and that's part of the problem that you need to be able to measure how far bends.
If I wanted to use it as a magnetic sensor instead, which I could do,
I could bring this magnet in here and you can see that it starts to bend.
You're using, oop there is snapped, and now I actually
have a better accelerometer than I had before at least, it's more sensitive.
Now you can more easily see that it bends.
Now, even with just eyeballing this you can see that there
is an accelerating field this way.
That's why,
if it was bent that way instead you would know that I had turned it upside down.
Take a look at this.
When I now engage it, I think that if you remember back to when I did it before,
it moves quite a bit faster than it does now.
So there's some things that happened to the statistics here when I make
it more sensitive.
I made it more sensitive to gravitational fields to just as a scale,
if you like, I made a more sensitive scale or
more sensitive accelerometer by doing this, but I also made it slower.
So that's the point that I want to make.
If you now switch back to my slides here then we can see that what
I've showed you is essentially the system that I've shown here in the figure.
There is a spring, that's the spring constant there k.
There's a mass that's moving on it's, I didn't talk about this, but there's some
damping, but you can certainly see that the swinging didn't go on forever.
So damped out, maybe they didn't damp out fast enough.
I'm looking at it over here.
Now, it's still vibrating, but it is, the vibration goes down,
so there's the damper, and
then there is something that we didn't really have in our experiment.
I asked you to just eyeball how much the thing moved, and
that's some kind of measurement for how much a mass is actually displaced.
So if I think of this as an accelerometer, if I modeled an accelerometer this way,
then I have a proof mass m that is going to be displaced by acceleration.
If I accelerate the whole system and
get a displacement of m going to, that gives me a force,
m times a, and that has to equal the spring force.
So I can calculate.
Once I just measure this displacement, I can calculate the acceleration
that the mass has been subject to by knowing the spring constants,
measuring the displacement, and dividing by the mass.
That's my measurement, and this is how accelerometers work.
This is how they work in your phone, this is how they work in your car,
this is the exact mechanism that is being used when an airbag deploys in your car.
So you have to sort of either live with more and just the simple formula that
tells us what the acceleration is if you have a certain displacement.
You also have to realize that there is a bandwidth associated with that,
we have showed you that, that once I made the displacement larger,
by putting more mass on my spring, I had a slower resonance frequency.
That's essentially meaning that I can't measure signals that are fast anymore,
I can only measure slow signals once I do that, so that's the tradeoff.
The tradeoff is shown here.
Well actually I should tell you one thing first.
What I asked you to do when we looked at the video was to just
eyeball the displacement in a MEMS sensor we don't do that.
We use some kind of adaptor characteristics to measure displacement,
and in our accelerometer, we use capacitive measurement.
So now you see that I have the same system I had before, but I added a little bit.
I added a little circuit here that just basically puts voltage on these plates,
across these three plates.
So there's a counter electrode down here, the mass has become an electrode, and
I have an electric field here.
I can now measure the distance between those two plates by looking at
the capacitance in this circuit, the capacitance between them.
That's a very, very good measurement.
I can measure very, very accurate in this way.
I can measure distances that are much more than the size
of an atom with this type of technique.
So, and this is part of my sort of small is beautiful argument, that can
really match a very small displacement, very accurately with this technique.
If I wanted to measure something that's large, and moves a large rate,
for instance your car is pulling up to a to a stop sign or a traffic device.
And then after a little while it's changed,
then it's measuring the position of your car magnetically.
That's too large distance, and so for
small distances very accurate measurements you use electric field.
So once you have that, you have the measurement of precision,
delta z, then you can calculate the acceleration, like I said.
And I can rewrite that in terms of that formula that I showed you for
the resonance frequency In this way.
So here, this is really the central design equation for an accelerometer.
And of course, like I said, it's not only the accelerometer,
it's always the other sensors and actuators too.
So unless you wanted to not measure the smallest possible acceleration in
the given bandwidth.
So you want to go up to certain frequency, there's certain signals that are so
fast you don't care, but they do care about certain signals up to a frequency f.
You could try to make that happen by
having very low [INAUDIBLE] and the very large mass.
And I would get a strong signal but I also would get a very low resonance frequency.
And if you take a look at that frequency, resonance frequency,
that's [INAUDIBLE] the square [INAUDIBLE] over the mass.
So that attempt isn't very good.
Making a good sensor by making a very weak spring and a large mass.
Well, it sort of works but it doesn't really work for
the frequency side we're interested in.
What we can try to do is to make a miniaturized accelerometer.
So now we have a very small mass, but we also then compensate by making a very,
very weak spring.
It's only really the ratio to that is important.
So small is okay.
It's okay to have a small mass, because you can also make it a weak spring and
it still remains in a good, small, measurable acceleration.
So what it really comes down to is [INAUDIBLE] is displacement [INAUDIBLE]
very accurately.
[INAUDIBLE], that's nothing to be good enough using capacitors if you measure
that very, very accurately.
But the key there is to make them so we [INAUDIBLE] small structures,
because they have excellent position sensitivity.
And you can just try any resonance frequency you want, because it's a ratio
of two different quantities of the spring cross in the mass that matters.
These lead us to matter of main argument over these last few slides.
Anyone who's used miniaturized sensors and you want to make
them as small as you can possibly make them and that's where MEMS comes in.
So MEMS stands for microelectromechanical systems and like I've said before,
these are systems or sensors that are made using integrated circuit technology.
This is something that started way back and Stanford was very central to it.
This is some figures from an article I read in 1983,
when it was published in Scientific American.
And James Angell and the other gentlemen on this
paper were here at Stanford at the time and they did some very, very cool things.
They wrote about this gas chromatograph,
which is this, I'm going to explain how that works.
This actually is not an example of something that's a mass in the spring.
It's a wafer scale integration, it basically
measure the composition of gases, the gas mixtures.
This one here in the lower right-hand corner is an accelerometer, then of course
here, you can immediately recognize the string right there and the mass.
So I'll know this is a spring with a mass on it.
So this is exactly what I just showed you.
And then here's another one.
This is a pressure sensor.
If you're measuring just static pressure if you just try to follow the weather or
something, then maybe the [INAUDIBLE] frequency isn't that important.
But if you use something like this as a microphone, [INAUDIBLE] and
many of you have these kinds of microphones in your cellphones.
Then again, you have your resonance frequency as the key parameter and
you have the same.
Sort of you have the same argument for making this as small as possible.
This was an incredibly powerful article for me.
This is where I did what I did later.
Three years after this came out, I was a staffer myself.
So and I lived in Norway when I read it.
So this was sort of life altering for me.
Another thing that came out was article by Kurt E Peterson.
In the particular figure that I pulled out of that article.
He had article was or the publication was called Silicon as a Mechanical Material.
And of course, that goes to the heart of what I'm arguing to, silicon is really it.
That is the best possible material to make sensors out of.
He solved, and I'm sort of reproducing that figure here,
a piece of work that was done by a previous student here at Stanford.
Roylance was her name, and she made some of the first MEMS accelerometers.
So these are, basically they're forefathers if you like
of the accelerometers that you have in your cellphone and in your car right now.
This structure as you see there and [INAUDIBLE] recognize.
If you look at this structure, that you have a spring and approved mass,
exactly the same thing as I've been talking about.
So, when we make MEMS, when we make microelectricalmechanical systems,
we use many materials.
Essentially if you go through the periodic table of elements,
almost all of them have been used in some fashion, either for
fabricating MEMS or being part of MEMS structures.
But then really the most important parts,
I'm not going to go through the whole list.
We have a whole large number of materials that we like to use and
that'd be okay to use.
The silicon is the sort of mainstay, the one we use always.
And the reason for that is that's it's stronger then steel,
lighter than aluminum, and it's single crystal.
Then, it can also be used in polycrystalline form,
a single crystal in particular, some very, very good properties.
And there's no other mechanical material out there that's anywhere like it.
If you think about construction and so forth, you think about steel maybe.
And steel can be single crystal, copper can be a single crystal,
but they never are when you are talking about constructions.
Only silicon is the single crystalline in mechanical systems,
and that's what makes it so unique.
So just a little bit about silicon,
it's in the group four in the periodic table of elements.
And therefore, it has the diamond like structure, same as carbon and
it crystallizes.
That means that there are some certain planes within silicon
that are more robust than others.
So if you expose it through chemical [INAUDIBLE],
some planes just won't etch as well as others.
And that means that you can make structures like these.
I'm showing you some structures here.
There you can make very well-defined structures that
are essentially terminating on the 11 1 planes.
These [INAUDIBLE] planes are more robust than the other planes.
And what that allows you to do is to machine very accurately in parallel, and
that's the key point.
You can make machine in parallel.
To think about a machine shop, you have a machine there,
you take a piece of aluminum or a piece of steel, and you machine it.
You do it at one at a time.
If you go back to that, that's a visual light processing technology.
These instruments they have a million little machines on their chip.
You couldn't possibly make them one by one.
So this idea of making them all in parallel and
making them by chemistry as opposed to by machining.
Machining by chemistry if you like, that's very central and
here's how you start that.
And here are some of those typical sensors.
One thing that was very popular for a long time was to use piezoresistive
sensors to do the measurement of displacement.
Actually, capacity before, this is again from that same paper that
Where the pressure sensor in the lower right here is from
that Scientific American article by Anschiel and his coworkers.
So this is one example of how you make a pressure sensor that is actually not
capacitive in this particular case but uses another transduction mechanism.
One thing that made a huge Impact on the silicon valley was using these things for
inkjet nozzles, so this is the basis of that transformation that happened within
HP, Hewlett Packard, when they became sort of a printer company for a few years.
This is a part of an inkjet printer.
Of course that was something that's maybe has come and gone but it was very,
very important for a long time.
So this is by machining things out of silicon, so
out of material that I think so highly of.
Another way of making micromachines is to deposit layers on
top of silicon and then shape that.
And this is the sort of canonical example of that.
This is a Combdrive that could be sitting in something like a timing circuit or
an exometer, and in fact, that particular
technology has been taken to extremes by a number
of companies and also institutions around the United States.
So Sandia in particular made a very, very sophisticated
surface micromachining process that you can make almost anything in.
So and
then of course surface micromachining is also the underlying technology for
that digital light processing technology that I already talked about a little bit.
Another aspect of fabrication that sort of leads up to modern day
micromachining in MEMS is deep reactive ion etching.
Some of the most seminal work in deep reactive ion etching for
MEMS is also done here at Stanford.
How it works is that when you try to etch into silicon you tend to sort of get
shapes that are not exactly ideal.
So if you take a look at that cross section in the upper left hand corner,
I started to etch into silicon, I'd get these slopes that were rounded side walls.
And what I could do then, and what happens automatically in this process,
in deep reactive ion etching, is that I deposit a polymer.
And then I etched a polymer at the bottom of that hole.
I executed a directional etch so that the bottom is exposed and
I can repeat the process.
And I get, instead of sort of getting a very large or rounded sidewall,
I get a sidewall that consists of these scallops, little scallops, and
they can be very, very small.
So essentially, even, I've done this.
I keep repeating this, this deposit polymer then etch,
deposit polymer then etch process, I get something like what I show here
in the bottom at right hand corner where I basically get straight side walls.
This was a huge first step forward,
because now we can shape things differently.
Now you were no long dependent on the crystalline orientation of our silicon.
Now we can shape it more arbitrarily, so you can do things like I'm showing here.
This is a very much used type of vapor in maps,
it's silicon insulator, what it means is I have taken a silicon wafer,
deposited oxide on it and then put a layer of silicon on top of that oxide again, so
that's where I'm showing on the top here.
And then we just take that DRIE and
just shape that top layer into whatever we want.
And then we remove from oxide underneath,
from where we want to let them have free stranding structures.
This is essentially the basis process for a large number of MEMS devices.
And here is sort of just one example.
This is close to 20 years old now, so it's not sort of the modern type necessarily.
And there is a couple of points I wanted to make here.
It's a very simple process.
Therefore the structure that you see is made in one mask in an SOI wafer,
and it has a very, very powerful functionality.
It's actually a fiber optic switch, this particular example.
The point I want to make is that it's way too large.
I said that's MEMS One of the fundamental reasons to use MEMS,
and particularly in the Internet of Things is that you're going to have so
many sensors, and that they are super small.
So, the question is how do we take this type of technology I talked about there,
the chemical machining, the surface micromachining, and the DRIE,
how do we combine those to make really small sensors?
That I think is where the futures are, very, very small sensors.
And here's one example, this is from my own work where we've been able to put
a large number of the third functionalities together,
this is an atomic force microscope that is so
small that it fits on the facet of an optical fiber.
A standard optical fiber is 125 microns, so
that's a little bit more than a tenth of a millimeter in diameter.
And the output, a fully functional atomic force microscope on top of it.
So that is sort of a tour de force in fabrication if you like.
But it also shows that we now have the technologies to go smaller.
One of the key technologies behind that particular development is shown here,
that's Hydrogen Annealing, so If you start out with a piece of silicon and
you print at low pressure in a hydrogen ambient, and
heat it up a little bit, you'll actually get sort of
a reconformation of the silicon, it will flow.
It still stay in sort of a single crystalline form but
it can make all kinds of very very interesting devices.
What I'm showing here is on the left of a photonic crystal,
on the right I'm showing that how we can actually use this to make boards,
I'm going to later in a little bit more careful detail later, one of in
the picture in the lower left hand side, no sorry, lower right hand side.
It's one of my favorites because you can actually see in those boards that
are created you can see the crystalline facets of the silicon.
So we are showing that this is in fact sort of a crystalline
material and even that these boards show this very clearly.
So just to show you, this is what I think is the basis for
a number of sort of very important MEMS sensors going forward,
sort of assess a technology that allows you to make more or
less arbitrary shapes of silicon and I'm demonstrating that here.
So we start here with a, following it numerically from one and then up.
There's a piece of silicon, we deposit a matching layer,
we pattern something into the non-matching level three.
And then we etch into the silicon.
So only one match so far, and in fact there can only be one match.
And then I do a little trick there in number five,
I cover everything conformally with a thin cell.
And then in six, I've continued my trick, I've now removed things from the bottom.
That's just a sort of an idea we got from the DRIE, right,
I remove things from, for example, surfaces.
The top surface doesn't matter,
which is already covered by such a thick patternating layer.
And then I go and actually the silicon with a directional etch,
and in number eight, I go through with a isotype with an isotropic aspect,
created these spheres for me, these spherical hollows.
And then I continue.
I just do the same thing again in nine,
directionally a bit further down I do the conformal coverage in tank,
I do the removal from horizontal surface [INAUDIBLE] 11.
I continue the action trial.
I do another, this time much longer.
As the [INAUDIBLE] catch in 13, and then I remove all the cultivation in 14 and
the I have that structure that you see there.
And then, some of the magic happens, because going from 14 to 15, what I've
done is that I've done the hydrogen anneal where I have re-flowed the silicon.
And that allows me to make this very, very complex structure.
It may be, this is a cross-section and sort of a cartoonish thing, so
I want to show you some SEMs of that same thing to really show you what it looks
like in real life.
So this is before.
This is at level 14 in the fabrication process.
Now we're going to cut it off so you can see what it really looks like.
There is this hole down in the silicon,
there is a spherical hole partway down, and then there's a void that
is continuous that stretches from hole to hole further down again.
At the top you can see it's just these cylindrical holes.
And now then we do the magic.
Like I said, now we do the hydrogen anneal, and then this is what happens.
So if you now see that those spherical holes that
I hide in the upper layer have turned in to voids and
they actually, the silicon flows on top of them and enclosed underneath them.
And it left two structures, it left these voids and they are separate and
it left a sort of continuous void underneath.
Now I really have, this is actually a very good pressure sensor.
We tested this in many different design.
Because that, Sector or the small, spherical voids on top,
it comes through a photonic crystal that gives me higher productivity.
So we actually use an optical readout, an optical interferometric readout to make
this into a very, very precise pressure sensor.
The whole structure is made right there.
I think that you would agree with me that there is very few limitations on what I
can make in terms of shapes with this.
And the really, really nice thing about it is that these are single crystalline
silicon, and they are extremely small.
And those two points hang together,
because by making things out of crystalline silicon,
I can make things very small and still have them be very, very robust.
I can control the process better so I can go through very thin films and so forth.
So this is a very, very, powerful nanotechnology.
Here I'm just showing a simplification of what I just showed you.
This time I forgot the, I forego making that upper layer and
I just make it void, so if you see that down there at ten I just made it void.
This is a pressure sensor by itself.
The diaphragm is now hitting on top of a reference cavity,
that is essentially very little pressure inside of the void that I created because
when we do the re-flow, the hydrogen anneal, that's very low pressures.
And then we end up with something at the very tip, defined pressure sensor.
And simplification is super important here, I believe.
You need to have things that are simple if you're going to go to the Internet and
things.
So that's why I love this particular pressure sensor.
It's just silicon.
And it has just a void in it.
And I can read it out capacitively like we talked about before.
How much that upper diaphragm has display, where it can measure its optic rate.
There is a number of different things I can do with that, but it's super simple.
That brings me to sort of the point I wanted to make about smart sensors.
Since I've been working with, like I said, in 1983 I got super excited about MEMS and
I decided to come to Stanford to study that.
And worked with these people, I never actually worked with James Angell,
but I did play golf with him a few times.
He's unfortunately passed away now, he was a great person.
But I worked with people here who worked with MEMS.
And one of the conundrums for MEMS is the idea that since your making
it from silicon, you should put some intelligence into the sensor.
And I showed you examples of that already by the Texas Instruments technology.
There's a set of switching transistors and underneath the mirrors that I showed you.
Analog Devices is a company that does really,
maybe around a third of them in commercial arts.
In capacity of exerometers and gyros.
And they also have integrated electronics
on chip and other examples of that.
There are few out there but not many because it is very difficult to do that.
So, then we were talking about the Internet of things where
the sensors are going to be a bit cruder so
you might have to go a much cheaper element than you can get with this.
Cross the same thing or estimate, reduce MEMS and CMOS together.
I don't believe that that is going to drive the Internet of things.
I think that what we really have to do is to do what I'm saying here at
the bottom to assemble things together.
Assemble the electronics together with very simple sensors to
make the simplest possible devices and systems that we can make.
So that sort of makes it back to the Internet of things.
So like the proverbial elephant, the Internet of things depends completely
on your perspective, or what part of the elephant you grab on to, right?
I'm sort of grabbing [INAUDIBLE] by the sensors that you have understood.
They are the most important part but that's, of course, my perspective.
I think that that's where the real world meets the digital world and
that's the most important.
Other people say no, no, no.
Come on, the sensors have been around forever.
What really is new here is the Internet and the machine learning.
And then of course what's exciting is what this can do for innovation and so forth.
What are we going to do with big data?
And then of course other people say no, what's really most important is how you
implement all this in the circuits and the embedded systems and so forth.
So exactly what the Internet of things is, and
what's most important, I think is a question that I can't answer.
My take is that the sensors are the most important,
those are the ones that enable things.
But I think I do can say a little about opportunities and the cool applications,
so let me have a stab at that.
One thing I've been hearing a lot about is that, and everybody is excited about it is
autonomous vehicles, particularly cars but it also extends to airplanes,
drones, underwater exploration, and aerial mapping and so forth.
So here's sort of my little animation of that.
So right now, the way most of the autonomous vehicles operate is that
they have a LIDAR, that stands for light detection and ranging.
And basically what I'm showing is a laser beam sort of scanning across the field
of view, registering things where things are, it's almost like a camera.
They have a little bit information because it can give you
a little bit of motion information and so forth.
And it also has maybe bad resolution at a distance.
But basically you're getting an image of what's going on.
And that's not what our eyes do when we are driving.
We do something quite different.
We are the environment, we don't photograph it,
sort of with a certain frame rate.
We watch areas of interest.
And we also are very good at picking up emotion.
And that's what we need to in sort of future self-driving cars.
So here's sort of what I think it should do.
It shouldn't just scan across, it should take a look.
There comes a dog running out, I have to look at it.
So that's what a human does.
You just look at the tree after all because the trees there,
they topple over and that's a problem if they move.
But mostly, they're standing still and they're going to continue to stand still,
sort of at least assumption.
So awareness is really what the Internet can teach us all about,
being aware of the environment.
One way to do that,
to get that functionality I just showed you is used phased arrays.
So this is one possible solution.
We are working with that,
the scanning mechanisms that allows you to jump in any sort of in your field of view.
Not scan, sort of get the full picture, at the same time you're
looking at exactly what's important, just like a human would.
And that's where some of the and
batches are going to come when you actually have a self-driving car.
I do think that there's a very good chance that it's going to operate a little bit
more like a human in terms of what it actually senses and
what it pays attention to.
And that's what this particular technology allows you to do.
This is a MEMS phased array.
I'm not going to talk too much about how it works but
it's essentially the same kind of structure again, right?
Mouth and the sprig.
Here's some other things that I think that are super exciting, but
also very troubling in some sense.
We have wearables and that's not really a big deal, everyone's wearing a Fitbit or
something like that these days.
Then you can ingest sort of diagnostic devices in pills and so forth.
That's a little bit more invasive.
And of course, I'm working in the horrible end of this being implantable sensors.
What I'm showing here in the upper right-hand corner is an implantable
microscope that allows you to follow cancer progression in animal models.
So this is sort of very invasive.
They can also give you a lot of new information.
And then the one on the bottom right is our srot
of implantable microphone, I should say.
I basically look at sort of acoustic fields in the body and how those,
what they tell us about the different diseases and so forth.
These are very, very exciting fields and
one of the people are really going into this examples here.
A young professor Aida Pru who's has actually put together some of this slide.
She is in the process of revolutionizing this, and improving all aspects of it.
And you will see more and more, in particularly, sort of the ingestible,
the implantable sensors are just starting out.
Now, so this is what they are and I think that you can expect to see more of them.
They've brought up some of the challenges and opportunities that they represent.
You're going to get, just more and more health records [INAUDIBLE] and
you need to integrate actually can really sort of get [INAUDIBLE] information.
That's a big data problem, and mainly machine learning problem.
Data security of course, is super important,
you don't want people to hack our informatiion.
But here's another thing, that I'm talking about here.
False positives, if you have a sensor that tells you
that you might have a symptom that is problematic, then you go to your doctor.
So the doctor's office is going to be full of healthy people, but
they are anxious about some device that they have that tells them something.
Now that, of course, if you ask doctors,
they will say that's going to be a huge problem.
That's going to be a nightmare, this is not going to work.
But you can't turn that around, because there's an energy there,
people care about their own health.
What we really need to get to is that, you don't really just go and
bother the doctors about false positives.
What we do is that we become an assistant to the doctor,
say somebody can actually work with them, the doctor on the team.
And I think that the Internet of Things and
sort of it goes to medical sensors are going to help us do that.
Now, so in terms of opportunity, if you an find the business model that allows
you to tap into that energy that people have about caring about their own health.
I think you will win big.
I don't know how to do that.
This has so many aspects to it, [INAUDIBLE] is only one of them.
So to end up,
then what do I see as the big opportunities of the Internet of Things?
And what are the cool applications?
I think that the Internet of Things, like I said, even if I can't really define it,
it's by awareness.
That's really one way to, if you want to use one word that
describes the usefulness of the Internet of Things, it's awareness.
It's going to lead to efficiency, safety, sustainability.
In transportation and healthcare, food in production,
transportation, storage, preparation.
You're going to get smart cities, smart homes, and
it's beginning to have a lot more awareness of what's going on around us.
And if you talk about from a company point of view,
if you want to sort of entrepreneurship kind of point of view.
It's going to be a lot of people trying to develop business concepts around this.
So you really have to be unique, you have to have your own secret sauce,
I think unique sensors is easy for me to think about.
How do you make the censors unique.
It's not that you can measure things that haven't been measured before, but you're
going to use them in different ways and you can integrate them in different ways.
You're going to communicate with them in different ways,
that's a unique [INAUDIBLE] that you need to develop.
You can also be unique on how you execute.
That's a lot harder, at least from my point of view.
And of course, people are going to win there too.
Part of the execution is how you actually do some of these things for
the circuits and the battery systems.
Have better user interface I think is super important.
We really need to solve the big data problem by having
machines take that over and present us with information as opposed to data.
Maybe the most exciting things about the Internet are things that can be
effective in so many positive ways.
If you work on that, you have sort of so
many opportunities to do good, and as you also do round, that's okay.
But I think that doing good is really,
really what makes me excited about the Internet of Things.
So with that, I'm going to thank you for this thing and
I hope that you have some questions.
And we're going to take those now I believe.
Yeah, so I will start by the question about limitations or on miniaturization.
The question is, is there any limitation for
miniaturization [INAUDIBLE] structure reaches the nanoscale?
And clearly there is such limitation, there are such limits.
Once you are getting down towards the sizes that are comparable
to sort of atomic distances or a separation [INAUDIBLE] material.
They have a distinct or very well-defined atomic
distance between the nearest neighbors.
Once you start to approach that course,
everything that I talked about becomes very different.
So I think that you can take many of the crosshairs that we have made and scale
them down into the hundreds of nanometers certainly and maybe tenths of nanometer.
But once we smaller than that, and of course,
that's where electronics already is, right?
So the manufacturing base for going to those kinds of sizes are there.
We can go there, I've read the technology has been developed for electronics.
I have a hard time seeing sensors that
were the overall sensor is less than ten nanometers.
That would be crazy, it sounds to me.
So maybe somewhere in the tens of nanometers where the limits are where we
had to sort of start doing things very differently.
Yes, so I think that sort of answers that question.
So the next question goes to this thing about integration.
Is it possible to etch MEMS sensors on the same silicon dye as an integrated circuit?
And that is possible, and there are many strong examples of that, and
I keep bringing it up this digital life processing technology of
Texas Instruments.
I think that's a tour de force in technology development.
It has some, Electronics sort of underneath and
then you have built MEMS on top.
So that's definitely one example of that, and
there's several other companies that's done the same thing.
I think that to do that, if you look at the Texas Instruments' Technology,
it's only rarely updated, because it's so
difficult to do an update of a technology like that.
It took a long time to develop.
I'm not going to try to hatch at a guess as to the total development budget, but
it was going to be in the hundreds of millions.
And that means that only a few applications can be reached,
can really have ambition to do something as complex as that.
So I think that for most Internet of Things sensors, you will not do that,
just simply because there are other ways that more cost effective,
with the type of diversity that we expect to see in the Internet of Things.
And then there's a couple of questions about reliability,
And so let me just read a couple of other questions like this other question.
Is there any mechanical wear out with time for
MEMS sensors and of course [INAUDIBLE] more things.
And the next question is along the same lines is that MEMS currently
undergo extensive testing during manufacture.
What is your current thinking on long term testing on MEMS that
are in current operation, question is on reliability factors.
So I'm just talking about reliability in general first.
There is a large number of MEMS sensors out there.
Actually we expect to reach a trillion sensors.
A very small fraction, that maybe one in a thousand or
one in a hundred is going to be on Internet of Things.
There are many systems that aren't going to be connected like that.
And they are from these sensors and been talking about billions of them now.
It's from an excellent reliability and it isn't strange,
because the smaller things are, the more robust they are.
And of course, can you also add that this is the best material
known to man in terms of purity and it's a clean crystal,
it's a monolithic crystal in many cases.
Long term stability is excellent for all MEMS devices.
Let me just take one sort of example, it's somewhat facetious, but
if you take a BMW with many people that says it's an okay car, right?
And you shake it and we'll find that the first resonance frequency is
somewhere in the tenths of Hertz.
That means that it it's really not that good.
You should take a MEMS device and shake it and you try to look for
their first resonance frequency, it might be in the millions of Hertz,
in the megaHertz, or at least in the hundreds of kiloHertz.
Basically what I'm saying is that any MEMS device or
any good well defined designed MEMS device is something on the order of
10,000 to 100,000 times more light than a BMW.
Now I am being facetious.
It does go to its reliability.
Now, there was a more specific question in there that had to do with how we test,
and that is difficult.
I think that it is clear that the Internet of Things is such an explosive route that
we are not going to test enough.
For some of these application is going to, like you have to do more testing.
As a general rule, I think that MEMS is very irreliable,
therefore you have to test for it.
The next question has to do with something I didn't touch on at all,
and that is about how do you power these sensors.
So the question is about energy harvesting method.
Are there any MEMS sensors that can be self-powered by harvesting ambient energy?
And, again, this is a big field, I didn't talk about it, it's very, very important.
If you're going to have sensors everywhere, having bad ratios course
possible, some of these sensors are using very little energy and
you can waste them up occasionally when you need to read the data.
And that could get you into very, very small, sort of power,
through average powers, so you might be able to,
on the even very small batteries operate for ten years.
But of course, what's eluded to here, well,
energy harvesting is [INAUDIBLE] very, very interesting.
So the most obvious is use solar cells and
things of that nature if you are operating in an environment where that makes sense.
Even an indoor environment has a lot of light energy, typically.
So you can combine that way through a rechargeable battery.
You can have something that essentially lasts forever.
I should put that in quotes because nothing lasts forever, but
at least you don't have sort of a power lifetime that's obvious.
There's also a number of developments that goes to
things like extracting energy from vibrations.
So if you have a pressure sensor in a car tire, and that's more and more common
these days, so that you know whether you actually have a problem with your tires.
You don't really want to have a battery in there.
But there's also a lot,
there's high energy environment because all the rotation.
So you should be able to, you might have a battery but
it's a rechargeable battery that recharges through that energy that you can extract
from the rotation that's going on.
So energy harvesting, that is super important.
And when you say that the MEMS sensors are self-powering,
that's quite a different question though, because now you're talking
about the sensor itself being the energy harvester, too.
And my general feeling there is that's better to separate those two functions
into two different devices and put them together into a real functioning system.
>> So we're at the top of the hour, so to be thoughtful of everyone's time,
why don't you take two more questions, Olav?
>> Okay, yeah.
So let's see, the next one, I haven't read it.
So let's see, reality of the job.
Now how do you see IoT devices catching brain waves down metabolism and
help with deep learning disease prevention other body cures?
That's actually more than one question, I think, but
let me try to take a stab at that.
There's definitely, and I did mention Ada Prune as one of the premiere
researchers in this field who, lots of people are interested in neurology.
And they're using both sort of popular genetics and
also a number of different kinds of probes.
Some of them are based on MEMS technology.
So the brain makes it sort of a little bit of a nebulous term for me but as neurology
and sort of action potential and things of that nature are being measured with
sensors and they will be part of the Internet of Things at some point.
Although now we talk about something that's very, very invasive.
So exactly when that kind of data will be part of the Internet of Things is not
obvious to me.
And some of the metabolism and so forth,
It's something that is much more straightforward, and
we have quite a few sensors that are sort of in a micro [INAUDIBLE] system.
So we do all kinds of things like drug discovery and so forth.
And there, there's a large number of MEMS sensors there.
Deep learning,
of course, I think this is disconnected from the first part of the question.
Deep learning is a super important part of Of
the internet of things I mention machine learning deep learning a variation
of that I'm not going to go into the details of the difference.
But the deep learning is absolutely essential for
dealing with the big data that we're going to get from all these sensors.
And so that part of questions,
how does this plane do [INAUDIBLE] prevention and other body [INAUDIBLE]?
Having more data must help but it's not all this how you do that.
This is really one of the key questions and maybe one of key challenges or
we could turn it around key opportunities of how do we actually more data to
make healthcare more efficient and hopefully also less expensive and
also you have better outcome.
Not obvious that has going to happen and doctors are petrified of it.
Yeah, so I think the last question, I'm concerned about people hacking into
self-driving cars and another large subject that cause harm.
What are the safeguards?
This initial course very well founded because I did [INAUDIBLE]
has been examples all ready of cars being hacked into and
people if they were malicious I think most of the examples we heard about.
How people do with the demonstration where they could have
done things like lock the wheel and stuff like that.
I think that this is definitely dangerous territory.
I want to take parts that we have the same.
This is actually something that needs to be studied very carefully.
Now, take the self driving car as an example.
I think that they have to be aggressive in employing them, even in spite of the fact
that I really don't have a good answer for this question.
And the reason for that roughly speaking,
40,000 people die in traffic in the United States every year.
If we can reduce that number substantially,
even if we are opening ourselves up for malicious hacking, if the total
outcome is that we reduce that number substantially and you have to go for it.
That's why I think you have to be aggressive.
But this question, of course, points to some of the most frightening things
around the whole Internet, and I don't know what the safeguards are,
other than being very vigilant and making the systems as hack-proof as possible.
And if we are to believe certain [INAUDIBLE], we don't even
have a hack proof electrical system in this country, right.
So, it's a very tough question and I don't really know the answer, but
in some cases we have to go forward without being 100% secure.
In other cases, the downside is so huge that we just basically cannot take a risk.
So maybe one way to answer the questions is we basically have to parse
the risk-reward, and go forward where it makes sense.
>> Great thank you Olav so much for a wonderful presentation for
taking time to answer all these attendee questions and there were many we even
couldn't get to which shows me how much interest there is in this field.
A reminder to all of the attendees,
recordings will be made available within a week and sent out to those who registered.
And we just want to thank you.
Thank you so much for taking the time to attend today and we'll see you at our next
webinar and hopefully in our internet of things graduate certificate as well.
Thanks so much.
For more infomation >> Stanford Webinar- From Smart Sensors to Smart Networks - Duration: 1:00:05.-------------------------------------------
[VOSTFR CC] BTS X GFRIEND ~ Family Song MV Smart School Uniform - Duration: 3:36.
-------------------------------------------
HARD WORK X SMART WORK - Duration: 3:34.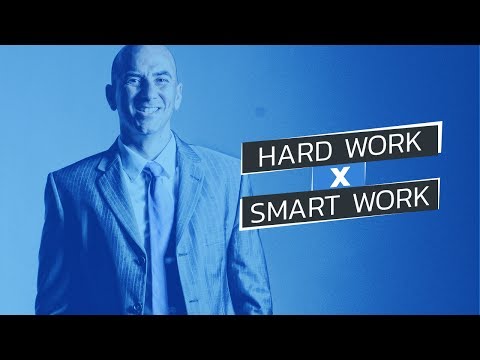
-------------------------------------------
Chereeki/Latec I3 Plus Smart Bracelet Unboxing Review and Test! - Duration: 3:43.
Hello there guys!
It's CoolFox over ehre and today, I'm bringing you an unboxing and review of the Chereeki
I3 Plus smart bracelet.
Taking a look at the back of the box, you have some features listed and opening it up,
you are greeted with the bracelet itself and a manual.
The manual can come in handy if you have 0 idea of how to operate the watch but other
than that, it is pretty much not needed.
The watch fits nicely around my hand and holding it down, powers it up.
Now initially it didn't have any battery so I had to charge it up.
The UI is minimalistic.
It;s all
in black and white no fancy colours or anything like that but it contains all that is needed
on the small watchface.
You go through the UI by pressing or swiping up and down.
It does have a heart rate sensor which is great to see at this price point and it does
work pretty accurately from what I tested out.
Some features are locked since you need a phone connected but I will do that later on
in the video.
You can also choose form these 4 watch faces.
The last watch face is the least favourite of mine but the first 3 do look quite appealing.
When you are greeted with an icon that contains info inside of it, you can long press and
it will come up with the information.
To get out of that screen, you have to swipe up from the bottom and that gets you back
to your initial screen.
The application has pretty bad reviews coming in at 2.8 stars.
After you register (which I do see as a bit pointless unless you change devices) you have
to input some of your information which enables the app to calculate your level of health.
After I registered and got it running it actually crashed and I had to put it back up.
Something to keep in mind is that you have to pair the watch through the app and not
through the bluetooth settings.
If done through bluetooth settings, you cannot connect to it.
The app itself is only used to track your history with the device you are using and
nothing else.
In fact you do not even need to install it.
Something that I found annoying is that the notification cannot be removed and stays there
unless you uninstall the app.
That instantly put me off of using the app and i wish they let you disable it.
I hope you enjoyed thsi video guys!
If you did, please leave a like and subscribe for more content.
This was CoolFox and I'm out.
Peace!
-------------------------------------------
Hong Kong Thinks Punishing Activists Is Smart | China Uncensored - Duration: 9:42.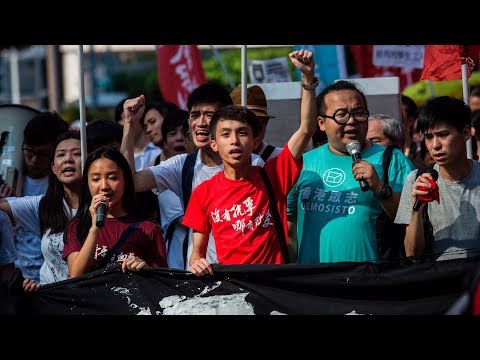
On this episode of Hong Kong Uncensored:
You go to jail.
You go to jail.
Everyone goes to jail!
Welcome back to China Uncensored,
I'm Chris Chappell.
The Hong Kong government has just made
a huge mistake.
Um, yes, but sending political activists to prison
is not exactly the mistake I'm talking about.
The mistake is:
If you're going to secretly undermine
the freedoms of your people,
you have to do it in secret!
Like, if you're coming home at 3 am
after sneaking out to a party:
get your friend to drop you off down the street,
and climb back into your room
through the window.
I'm just saying.
That's just common sense
and is not based on personal experience, Mom.
Anyway, Hong Kong authorities
are still kinda new at this,
which makes them inexperienced and foolish.
Because when the Hong Kong court
sentenced these three political activists
to prison on August 17,
it really let the cat out of the box:
The Hong Kong government
is undermining the freedoms of its people.
Of course, Hong Kong Chief Executive Carrie Lam
defended the move.
"Rights and freedoms of Hong Kong people
are protected under the Basic Law."
Ah yes, Basic Law.
That was the agreement the CCP and the UK
made ahead of the 1997 handover.
Under the One Country, Two Systems principle,
the Basic Law guarantees Hong Kong
a variety of rights and freedoms—
even rights and freedoms denied
to people in the rest of China.
Anyway, go on Ms. Lam.
"But the exercise of these rights and freedoms...
is not without limit."
So what limits did three dangerous criminals violate?
Well, Joshua Wong, Nathan Law, and Alex Chow
were influential leaders of the 2014 Umbrella Movement.
"The 2014 protests—
known as the Umbrella Movement—
were triggered after Wong and his colleagues
stormed the city's government headquarters.
They were later found guilty of unlawful assembly
and were sentenced to community service."
Just to clarify,
"storming government headquarters" in this case
meant jumping police barriers
in front of a popular protest location
outside the central government offices
known as Civic Square.
Anyway, go on.
"But that wasn't enough for the department of justice
which applied for a review....
Joshua Wong will spend the next six months
behind bars."
So Joshua Wong and the other two less famous guys
were sentenced to community service.
Which they served.
And then the court decided to sentence them again—
this time to 6 months,
8 months,
and 7 months respectively in prison.
Why?
Well, the Hong Kong Department of Justice
decided that the punishments weren't harsh enough,
and that they wanted a review of the sentences.
Of course Hong Kong Chief Executive
Carrie Lam will assure you,
Beijing had nothing to do with the decision.
"Our courts are exercising judicial powers independently,
free from any interference.
So any allegation that in these particular cases
that judges in the Court of Appeal
have made decisions under political interference,
again, are totally unfounded."
That's like when you sneak out at night
and then try to convince your parents the next day
that the lump in your bed that night
was you all along,
and totally not the pillows from the guest bedroom.
What's interesting here
is that Lam defends the judges
as being politically unbiased,
but there are signs that the Justice Department itself
wasn't politically unbiased when deciding
to "review" the sentences.
For one, it's highly unusual for them
to review the sentences in the first place.
There are reports that Secretary of Justice Rimsky Yuen
overrode his own prosecutors
in order to get those harsher sentences—
even though his prosecutors didn't want to do it.
Interestingly, Rimsky Yuen is also a member
of the Chinese People's Political Consultative Conference,
the terribly-named rubber-stamp advisory body
to China's rubber stamp legislature.
Being in the CPPCC
is a marker of status and influence.
And you can get kicked out
for not toeing the Party line.
But I'm sure he made his decisions
about the Hong Kong activists
completely without any political agenda.
So when the courts handed down the prison sentences,
their ruling talked about the importance
of deterrent sentences.
That is, by severely punishing these three people,
they would make Hong Kongers not want to protest...
sorry, I mean, "unlawfully assemble"
in the future.
Which worked incredibly well.
In fact, the sentencing reinvigorated the movement
for rights and freedoms in Hong Kong—
with over twenty thousand people taking to the streets.
There's more uproar over this than for other recent events
that eroded Hong Kong's freedoms.
Like allowing Chinese police to patrol in Hong Kong.
Or saying the joint declaration Hong Kong Basic Law
is founded on no longer has meaning.
Or kicking out democratically elected
pro-democracy lawmakers.
Again.
As you can see,
the Chinese Communist Party's influence over Hong Kong
has been getting really blatant lately.
So the question is why?
This carries serious risks for the CCP.
It clearly shows that the One Country Two Systems policy
that the CCP promised the UK
is being thrown out the window.
And that makes it hard for anyone
to trust the CCP about any agreement.
As Financial Times puts it,
"If a bilateral treaty,
signed by two heads of state
and registered with the UN,
can be unilaterally ignored,
what is stopping China from trashing
any other treaty it does not like?"
So why would the CCP take this risk?
Well, one factor is that the CCP
doesn't care as much as it used to
if the lack of independence
hurts Hong Kong's economy.
In 1997,
when the UK handover happened,
Hong Kong made up 20% of China's GDP.
It was also the only real way for China
to access Western investment.
But now, 20 years later,
other parts of China have grown so much
that Hong Kong's contribution to China's GDP
has fallen from 20%
to just 3%.
So why bother appeasing Hong Kongers with pesky freedoms
unless those freedoms have limits?
Another factor is that the government of the UK,
and every other country,
still wants to do business in with China—
even if they're not super happy
about this latest move.
I mean yeah the CCP is terrible
and breaks its promises,
but...cha-ching!
So put on that awkward smile
and move on.
And here's another factor:
After the 2014 Umbrella movement was over,
many of the young people
who participated in the protests
tried to continue to change the system from within.
For example, Joshua Wong and Nathan Law
founded a political party so they could
officially join Hong Kong's democratic process.
Nathan Law even won a seat
in the legislature last year,
along with many other new
pro-democracy lawmakers.
Unfortunately, Law was kicked out of office
last month for adding a Gandhi quote
while taking his oath of office.
That's another topic.
But now, their long prison sentences
trigger a rule that disqualifies them
from running for office for the next five years.
So the Hong Kong government
is effectively shutting them out of the political process.
It seems like a side effect,
but I think it's actually one of the main reasons
the Hong Kong government did it.
By disqualifying and shutting out pro-democracy legislators,
the pro-Beijing government is more likely
to be able to pass laws that the CCP wants,
but Hong Kongers have resisted for years,
like the patriotic education law,
or Article 23,
that anti-subversion law that would let police
search people's homes without a warrant.
But here's the thing:
This move is extremely stupid!
What happens when you force people
to operate outside the system?
When you show them that protesting
is the only way to prevent the government
from stomping all over your rights
in order to appease the Communist Party?
You get this!
Twenty thousand angry people protesting.
And we're probably going to see
more protests in the future—
because the Hong Kong government's decision
tells the people one thing clearly:
Civil disobedience is now the only way to make
effective political change in Hong Kong.
Now, as for Joshua Wong,
Alex Chow, and Nathan Law,
they've already started their prison sentences,
although their lawyers say they will appeal.
Hopefully prison won't harden them too much.
Joshua has already shaved his head
and talked about taking graphic design classes.
Good luck, kid.
Now, I personally admire these guys,
and for viewers who feel the same,
why don't you head over to Twitter,
and send them some words of support
from the China Uncensored 50-cent army.
Their twitter handles are in the description below,
and their accounts are being managed
by friends while they're in prison.
Don't forget to tag @chinauncensored
so I can see your tweets as well.
And as always,
let me know what you think
in the comments below.
Coming up after the break,
if only getting political prisoners out of China
were as easy as getting money out.
When I was in Hong Kong last December,
I was lucky enough to sit down
with Joshua Wong for an interview.
We talked about his plans
for the future of Hong Kong,
and we even sang a song of angry men
from my favorite musical, Les Mis.
Of course, this is before I realized
Joshua Wong was a dangerous criminal.
So click here on the left
to watch that interview now.
-------------------------------------------
How Poop Shapes the World - Duration: 3:55.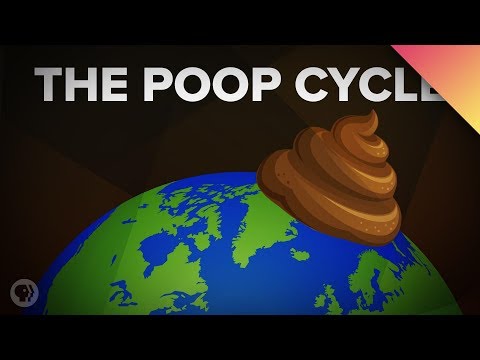
[MUSIC
Drop a plastic bottle in the recycling bin, and it might one day become something totally
new.
But the stuff we drop in the toilet?
We don't think about number two becoming anything.
But all over nature, the waste of other animals re-enters the great circle of life.
Here's how poop shapes the world we live in.
[MUSIC]
Viewed up close, corals are tiny tentacled animals.
But those living polyps sit on mountains of coral skeletons made of calcium carbonate.
When parrotfish eat coral, the bony bits they can't digest make their way back to the
sea floor.
In other words?
Parrotfish poop sand made from coral.
We're talking one metric ton, per fish, per year.
Enough to make up a big part of some of our favorite beaches.
Near the ocean's surface, marine algae absorb sunlight and carbon dioxide to make energy
and oxygen.
But like terrestrial plants, they need nutrients like nitrogen and iron to get the job done.
Giants like whales and whale sharks answer nature's call in a big way, pumping out
more than 50,000 liters of nutrient-rich waste in a single go.
Clouds of whale poop carry 10 million times more iron than seawater, so where whales "go"
phytoplankton thrive.
And when those plankton die, the carbon stored in their bodies piles up on the ocean floor,
eventually becoming things like shale and oil.
Phytoplankton are so small that billions can fit in a bucket of seawater.
But altogether, they absorb millions of tons of carbon from the atmosphere and give off
over half of the oxygen we breathe.
Much of the ocean's other waste falls to the deep sea in clouds called marine snow
where it feeds animals like the vampire squid.
But the poop elevator doesn't stop at the basement.
Deep divers like sperm whales hunt squid near the sea floor, then return to the surface
to breathe… and poop, recycling nutrients from the abyss
and starting the whole cycle over.
Ocean poop won't cure climate change, but without enough recycled nutrients, dying plankton
can disrupt the whole ocean ecosystem.
One plankton die-off during the Cretaceous period may have had a hand in oceans losing
their oxygen about 94 million years ago, causing a mass extinction that lasted five hundred
thousand years.
Poop can even move nutrients from the ocean, onshore.
Birds that feed on fish airdrop fecal fertilizer when they fly back over land.
Ancient reptiles like dinosaurs and pterosaurs would have done the same thing.
Enriched soil allowed plants to flourish like never before, and their seeds were spread
far and wide by the "movements" of monstrous fruit-eating mammals.
Without those prehistoric poopers, the scat-egories of lifeforms we see today may have looked
very different.
Some plants still struggle since the animals that used to carry off their seeds are now
extinct.
Passing an avocado pit is pretty painful if you're not a giant ground sloth, which you
can hear more about in this video.
Plenty of modern animals are still carrying the load, though.
After clearcutting rainforests, fruit-eating bats and birds can act like flying Johnny
Appleseeds.
Where they poop is where new rainforests grow.
Down in the soil, a typical earthworm population recycles around two tons of organic matter
per acre every year.
All this waste keeps terrestrial ecosystems healthy from top to bottom.
So from the forests to the oceans, we know our planet's lungs breathe easier thanks
to poop.
And that means we do too!
Stay curious.
-------------------------------------------
Little Bitty Spaciously Smart Tiny House For A Family | Tiny House Listing - Duration: 2:38.
LITTLE BITTY SPACIOUSLY SMART TINY HOUSE FOR A FAMILY
-------------------------------------------
Smart Attack - Duration: 4:23.
-------------------------------------------
Smart Forfour 1.5 CDI PULSE Airco Nieuwe APK Inruil mogelijk - Duration: 0:59.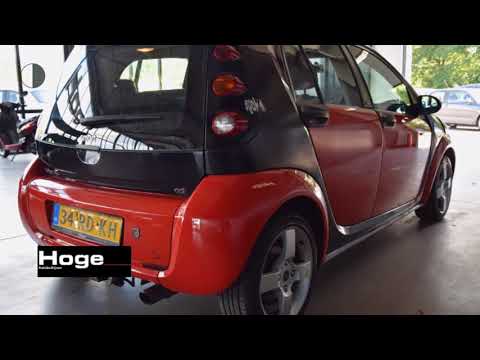
-------------------------------------------
Smart Forfour 1.5 CDI PULSE Airco Nieuwe APK Inruil mogelijk - Duration: 0:57.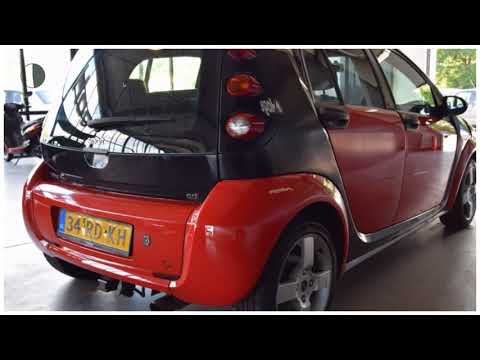
-------------------------------------------
Unboxing and test of LG 32LB650V ZN Smart Cinema 3D TV - Duration: 19:52.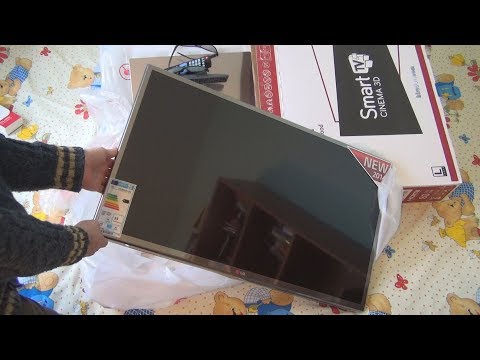
Hello,
today I will unbox a LG (Life's Good) Smart TV Cinema 3D.
This is the whole package
as you can see it's quite heavy.
It is 80 cm or 32 inch.
The model is written here:
It is 32LB650V.
It is HDMI,
Smart TV, Cinema 3D, Triple XD Engine,
this is the size.
Only for container.
Here I have some legal information.
This is the backside.
32LB65
This is the options
Triple XD Engine, WebOS, Smart Share, Cinema 3D, Smart Sound Mode.
Label.
Okay, now
let's open.
What we have inside.
Power cord.
Holder.
Styrofoam.
Another holder.
So, actually this is the
base, and this is
what we'll use
for mounting the TV set.
Still many things inside.
Remote control.
3D glasses
and manual.
Quite heavy manual.
This is the ... remote control.
No batteries inside.
Holder, so you can not
lose the back cover.
What's this?
Another holder.
Some screws - one, two, three, four screws
and batteries.
More screws.
Let's put
the batteries
inside the
remote control
I'll leave it for a later use.
Smart TV tag on.
3D glasses.
One pair.
Basic 3D glasses.
Another pair.
The same - black.
And
here we have the manual.
Wow, big manual.
No CD inside, only paper.
Many languages.
Okay, here we have only
left inside
the display.
Ta-dan.
3D Cinema.
Wow.
It looks gorgeous.
It is new.
2014 model.
LG.
Energy class A. Unfortunately, not class A+.
55 kilowatts per hour,
38 watt.
Let's take out this.
Bags and
use the power cord
to switch it on.
Nothing left inside the box.
So, let's take it out.
Aha, found it,
the power cable.
And now boot it for first time.
Cool, direct
power cord to the screen, not
an adaptor.
Not very long power cord, but for our
goals it will be enough.
This is the back.
I put here the power cord
and I have
many slots - one
external speaker
H/P out
A/V in-out.
SCART
Video
Y, PB,
Component In AV,
PR,
L-Mono, Audio,
Satellite IN,
Antenna cable IN,
and for DTS patents some text and CR
65227506.
Here I have the model
listed again - 32LB65OV.
This is for the holder.
Here I have holes
for mounting it to a wall.
It is not that heavy.
Let's plug in.
Take the power cord.
Power ON.
It works!
Life's good.
This is first boot of this screen.
It is loading now.
So, I shall press up?
Or okay.
Here we go!
Where are you?
Okay.
English and country United Kingdom.
Okay.
Down.
Default settings.
Let's choose some country - France.
Okay.
Okay.
There you are! Your TV has been located.
Internet connection type.
Let's turn the sound up.
Skip this step - okay.
Connecting your TV to the Internet provides a lot of benefits
including access to streaming content and handset TV listings,
web browsing and automatic software updates.
Don't connect.
This is just for this unboxing and unpacking.
Get ready to watch TV.
TV equipment and all your additional
devices now you will be able to watch whatever you want.
How do you get your Signal?
Select.
Is this for home use? -Yes.
Get back to get connected.
And now what shall I do?
Okay, okay.
Why I can not go down?
Back.
Uhm, how to?
Aha,
Set-Top Box, Set-Top Box only.
Or TV only.
Set-Top Box + TV.
Okay.
Now go down and okay.
Password is default.
Again.
Oh, why it is asking again?
Save.
Strange, okay lets try
four nines.
Confirm your TV password - one, two, three, four
types nine.
Skip this part.
I'll do this later.
HDMI.
Others service providers.
Set-Top Box manufacturer.
Skip this step.
Are you Sure? If we fix it now, you'll get all the benefits right away.
Yes, skip.
Antenna, cable, satellite.
I'll test this later.
Next.
Why it is not working?
Mhm, next.
Start digital only search.
Now it is searching.
No programs found.
Skip antenna TV.
Done.
Legal notice.
In French.
General terms.
Select all.
Agree.
If I can only select it easy.
Select all.
Additional services.
Agree.
Done.
Yay, you're all done. Now let's watch some TV.
Thank you. That's it. I will test the other features later.
-------------------------------------------
[자막] Esri UC 2017 Smart Dubai - Duration: 19:58.
-------------------------------------------
Smart fortwo coupe Micro Hybrid Drive Black Limited - Duration: 1:08.
-------------------------------------------
Mitsubishi Space Star 1.0 Intense+ (Bluetooth carkit / Smart Key) - Duration: 0:54.
-------------------------------------------
Everything You Need to Know About Fitbit's New Ionic Smart Watch - Duration: 2:35.
Everything You Need to Know About Fitbit's New Ionic Smart Watch
Fitbit has released what seems to be its most important product of the upcoming holiday shopping season: a smart watch it calls Ionic.
The product joins the companys existing portfolio of fitness gadgets, among them clip-on trackers like the Zip; bands like the Flex, Alta, and Charge; and fully featured watches like the Blaze.
The Ionic appears to be Fitbits new flagship wristwear, and with good reason. Heres a look at the device.
The Fitbit Ionic manages to fit—no pun intended—a GPS sensor, a heart rate sensor, a multi-day battery rated at more than four days, a Near Field Communications (NFC) chip for contactless payments, enough storage for music (plus Pandora support and Bluetooth headphone support), and water resistance into a device thats not much thicker than conventional wristwear.
Its also fully featured in terms of software.
Ionic offers personal coaching software that creates personalized workouts (its smart enough to recommend yoga after a tough workout, for example), guided breathing (for all that yoga), so-called SmartTrack intelligence that can detect your activity and adjust tracking accordingly, and—thanks to its water resistance—swim tracking that covers laps, stroke style, and calories burned.
Plus, its notifications work with phone calls, text messages, and calendar appointments.
The standard Fitbit Ionic is $299.95, a cool hundred more than its Blaze watch sibling and twice the price of the popular Alta HR band. It comes in three colors: a dark gray, a light silver, and a gold.
Theres also a special edition/designer Adidas model of Ionic, though its price has yet to be released. Look no further than the second generation Apple Watch for Fitbits stiffest competition.
Starting at $269, the chiclet device—which comes in dark gray, light silver, rose gold, and classic gold, with a bevy of additional options for extra cost—has GPS, a heart rate sensor, water resistance, personalized workouts, and guided breathing.
Garmins VivoActive HR GPS watch is also a popular alternative. Like the others, the rectangular device has GPS, a heart rate sensor, water resistance, and coaching software.
Its battery lasts much longer—approximately eight days in watch mode, Garmin says. It costs $249 but commonly sells for $199.
Finally Samsungs Gear Fit2 watch—thought its really a band—offers GPS, a heart rate sensor, water resistance, workout software, and a battery that lasts less than a day.
Its also the cheapest of this bunch at $189, though you can get it for less than $150 if you look.
So how is the Fitbit Ionic in the real world? Look no further than Fortune senior writer Aaron Pressmans first impressions. Wearing the Ionic seems like a statement that youre into fitness, that you like gadgets, or maybe that you just love Fitbit, he writes.
But unlike the Apple Watch or some of the new luxury brand Android Wear watches, the Ionic seems like less of a fit in a business meeting or at a fancy dinner party..
Không có nhận xét nào:
Đăng nhận xét